Grant focuses on using crowdsourcing to help analyze big data and solve problems
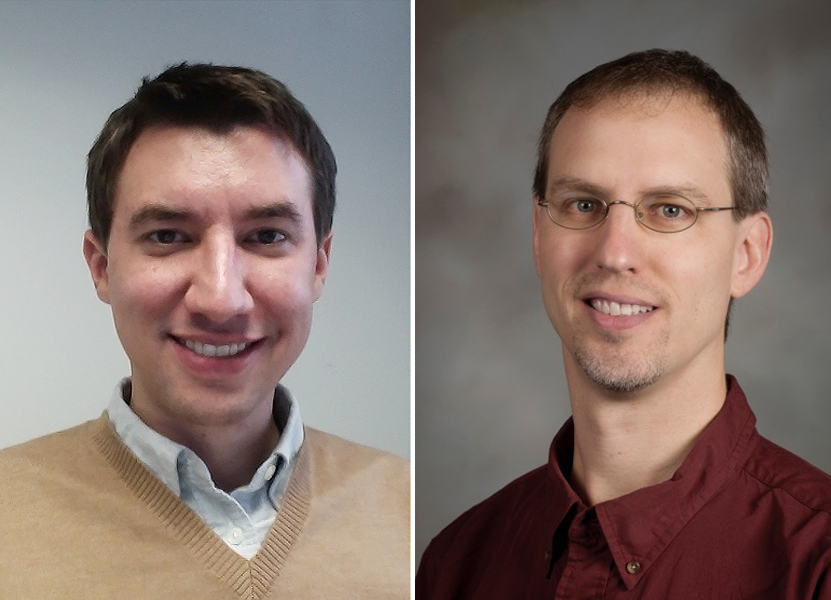
Big data, the almost endless growth of information available today to individuals, businesses, and government agencies, is often a challenge "to make sense of because human cognition, while remarkably powerful, is nevertheless a limited resource," said Kurt Luther, assistant professor of computer science at Virginia Tech.
For example, if an individual analyst is working on a complex task such as identifying a threat to national security, most advanced machine learning techniques do not have the needed capabilities to solve the problem. Neither does crowdsourcing, Luther added.
Crowdsourcing, in this sense, means soliciting contributions of data from a large group of people, most of whom are online users.
Luther and Christopher North, professor of computer science and associate director of Virginia Tech's Discovery Analytics Center, have designed a series of four experiments they said will enhance crowdsourcing as a "promising technique for applying human intelligence to problems computers cannot easily solve."
The National Science Foundation is supporting this work with a $500,000 grant over three years from its cyber-human systems program area.
Luther, the principal investigator on this project, said the challenge is to "understand when crowds are more useful than computation" in the attempts to make sense out of a problem. The second task is to determine how to provide crowd investigators with sustained complex lines of inquiry and not spend their limited amounts of time pursuing dead ends.
"We are in the midst of a data deluge," Luther quipped. "
How can an analyst sort through so much available information?
Luther and North, who are both faculty members with the Institute for Creativity, Arts, and Technology, think they have the answers with a novel concept they developed in computer science called context slices. The method uses a combination of human and computational guidance to give crowd workers only the information they need to complete their assigned tasks.
They will use their prototype in a series of experiments that are aimed at making better sense of big data with the simultaneous use of crowdsourcing. "Ultimately this system design will be a major step towards realizing our longer-term vision of developing powerful software tools to augment human intelligence and sense making," Luther said.
As an example, Luther described a situation where an analyst working in intelligence might be investigating three different individuals suspected of being involved in a terrorist plot. After collecting a large stockpile of potentially relevant documents including police reports, depositions, and surveillance footage, the expert can launch the context slices software program and sift through and categorize related documents.
The software will begin seeking potential connections between the suspects using both computational and crowd based techniques.
Prior work conducted by Luther and collaborators Steven Dow, Nathan Hahn, and Niki Kittur at Carnegie Mellon University showed how crowd workers can synthesize information from diverse sources gathered online to produce useful overviews. This work led to Crowdlines, a crowdsourcing system that generates an overview of a domain of knowledge, such as major topics in psychology, and allows users to easily identify the more common perspectives or idiosyncrasies across a diverse range of sources.
"From our earlier promising results, we conclude that crowdsourcing offers great potential to augment an individual's ability to make sense of complex and unfamiliar topics," Luther concluded.
The computer scientists plan to develop a new graduate course on social computing and analytics that will study the issues associated with big crowds for big data.